Current state: retail technologies not built for fresh
Until now, ordering and replenishment technologies have leveraged a system architecture originally designed for Center Store/General Merchandise. This approach consists of combining an intelligent demand forecast (using data science, machine learning, etc.) with rigid calculations of perpetual inventory, planograms, and safety stock in an arithmetic calculation to generate the order quantity. Accordingly and by design, they require perfect data accuracy to function. If any inputs have errors (i.e. PI), the system will generate bad orders.
As a consequence, when applied into Fresh departments, these technologies critically underperform. This is because fresh data is inherently incomplete and error-ridden due to constantly changing variables and unforeseen edge cases.
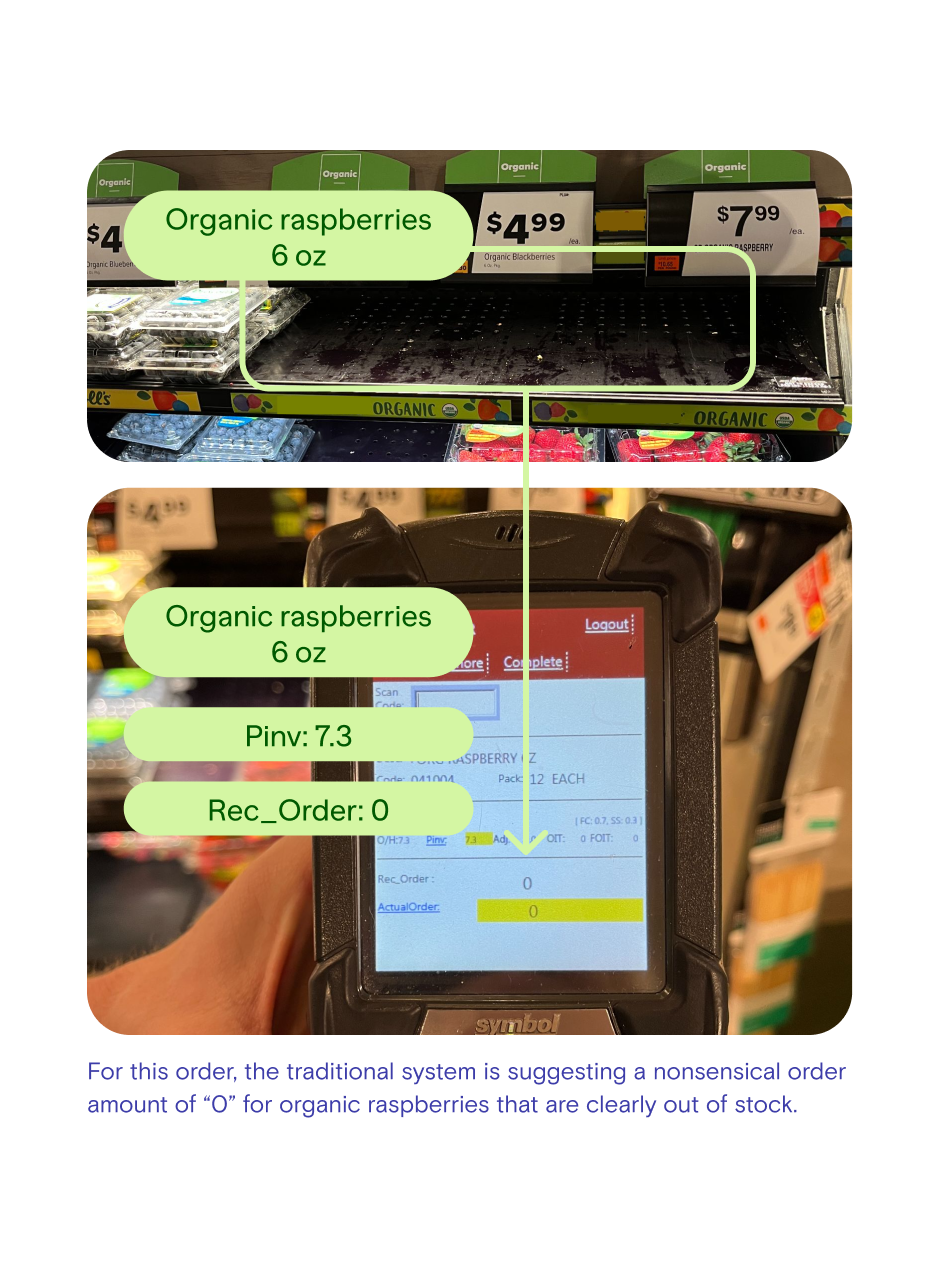
For example, a few misscans at the register immediately throw off a perpetual inventory model and misinform the next demand forecast.
The only mechanism that retailers have to improve performance is to invest excessive store labor and IT resources to manually keep the data accurate. Nevertheless, the constant edge cases of fresh cause perpetual inaccuracies that degrade end-user trust and ultimately lead to lost profits via excessive shrink, out-of-stocks, less fresh food, wasted labor and more.
To date, the leading approach to building a more effective ordering system has been to build a smarter demand forecast. But in fresh, while having an accurate demand forecast is required, it is wholly insufficient to be successful. In order to be effective in fresh, a system must assess the available data with the expectation of imperfection (which is inherent to fresh goods) and apply techniques jointly across inventory estimation, demand forecasts, and order recommendations to understand and adjust for the degree of uncertainty within the data.
A new paradigm: the Afresh approach
The Afresh Platform is an interconnected suite of solutions that leverage the latest innovations in AI and Machine Learning to optimize decision-making across critical functions of the fresh supply chain: store ordering, inventory, merchandising, operations, DC procurement, and more. We have developed a fundamentally different system design that intelligently navigates incomplete and imperfect information to drive optimal outcomes across fresh departments.
Our proprietary technology was developed by our founding team out of the Stanford AI/Machine Learning Ph.D. program. This novel approach to AI boasts accolades from leading conferences (ICML and NeurIPS) and validation from usage in other highly dynamic applications such as the development of autonomous cars. A few examples of this state-of-the-art approach include:
Handle messy data
Afresh expects and plans for inherent errors in fresh data. Our system applies AI techniques to model all inputs probabilistically (inventory position, demand, shelf-life, etc). By doing this, Afresh is able to understand the level of uncertainty within the data and derive accurate insights without requiring excessive manual labor or IT overhead to maintain perfect data hygiene.
Accounting for all fresh variables
As opposed to a traditional system that was built for non-perishables, Afresh accounts for all of the necessary variables that influence Fresh ordering. This is critical in order to optimize your inventory, demand, and order recommendations. In addition to the variables considered by traditional systems such as sales, shipments, pricing/promotions, and discards, Afresh accounts for a wider set of data such as item perishability, changing display sizes, weather, random weight (e.g. - bought in weight, sold in eaches), and substitutions/cannibalization among many others. With Afresh, decisions are made with a full picture of all the key variables that influence Fresh departments uniquely.
Decisions, not just forecasts
As opposed to limiting its use of Machine Learning to forecasting like many vendors do, Afresh actually employs Machine Learning across all inputs, and then combines these inputs in an intelligent decision-making engine. This allows Afresh to account for uncertainties and generate order recommendations with unparalleled accuracy – resulting in truly profit-maximizing order recommendations.
Modern, easy-to-use tools
Afresh intuitive tools mimic existing fresh department-specific processes and behaviors. They are flexible to edge cases, and prioritize end users’ labor time to provide focused input on select items for which the system’s confidence in the data is too low. This ensures trust and adherence by design rather than force – as evidenced by our average 94% recommendation acceptance rate across ~3,000 stores. It also creates a perfect balance of human instinct with all of the necessary data to drive the best decision.
The value that Afresh is driving with its partners would have been the single biggest return on IT investment I oversaw during my time as the CEO of Ahold USA. And that’s just in produce, let alone their entire solution across all of fresh.
-James McCann, former CEO of Ahold USA & Afresh investor
Impact
The outcome of this fresh-first approach is a lightweight system that fits seamlessly into fresh operations, empowers store team members, and generates recommendations with unparalleled accuracy. Across 3,000+ stores, the results speak for themselves. On average, stores saw a 3% sales increase, 25% reduction in shrink, 7% faster inventory turns, 94% adherence rate to order recommendations, an 80% decrease in out-of-stocks, and an impressive 20% improvement in labor efficiency.
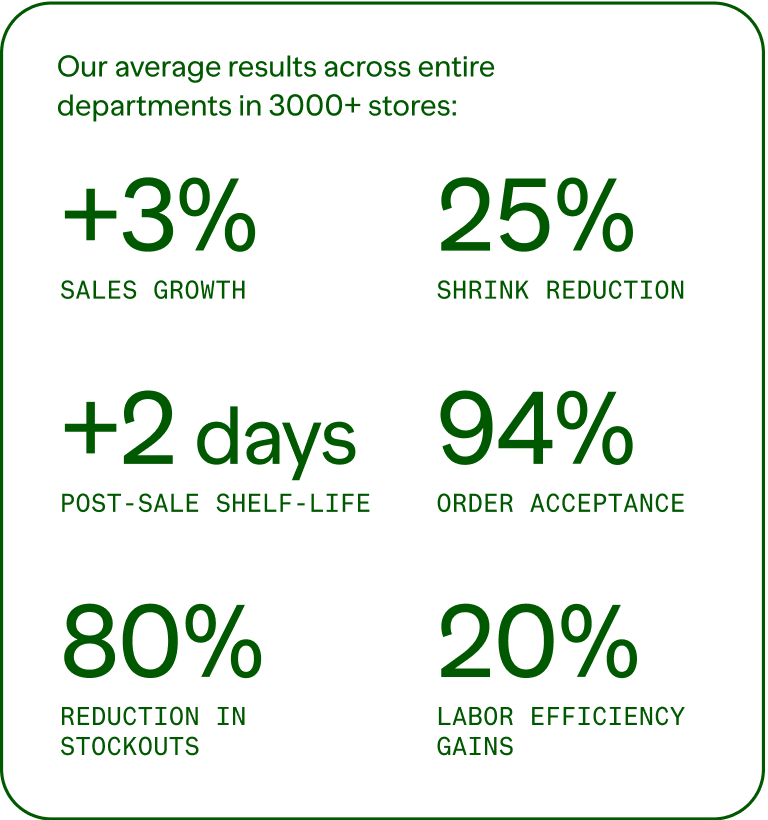
These are not just “up to” results that some vendors caveat in the fine print. These are demonstrated across our portfolio of partners including companies like Albertsons Companies, WinCo Foods, Cub Foods, Heinen's, Lowe's, Bashas, The Save Mart Companies, and Fresh Thyme Market.
We chose Afresh over other solutions because of their modern cloud-based architecture, non-reliance on perpetual inventory, and the ease of integration. We want to transform our fresh departments, and Afresh is the best for that job.
-Luke Anderson, CIO of Cub
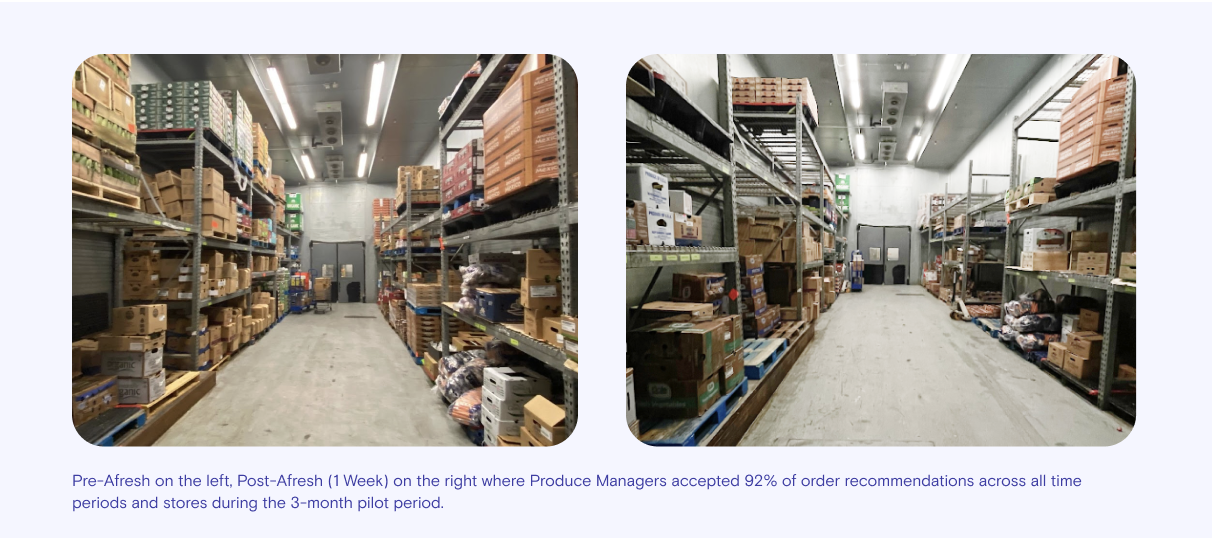